Pharmacovigilance 2.0: Next-Generation Technologies in Safety Database Analysis
- Chaitali Gaikwad
- Apr 20, 2024
- 3 min read
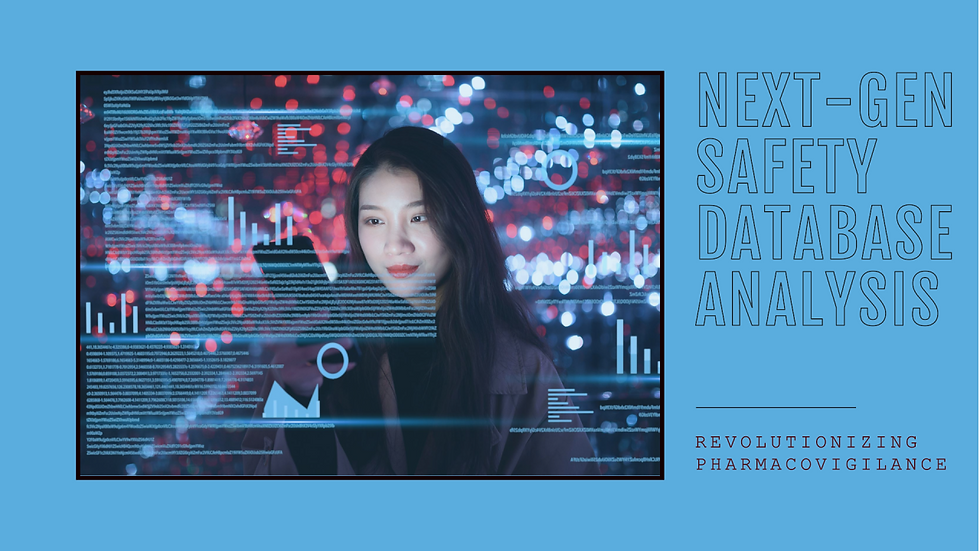
In the rapidly evolving landscape of healthcare, pharmacovigilance stands as a cornerstone of ensuring medication safety. With the advancement of technology, the pharmacovigilance field is experiencing a transformative shift towards Pharmacovigilance 2.0, characterized by the integration of next-generation technologies into safety database analysis. These technologies promise to enhance efficiency, accuracy, and timeliness in identifying adverse drug reactions (ADRs) and ensuring the overall safety profile of pharmaceutical products. In this blog, we delve into the key advancements driving Pharmacovigilance 2.0 and their implications for the future of drug safety monitoring.
1. Artificial Intelligence and Machine Learning
Artificial intelligence (AI) and machine learning (ML) algorithms are revolutionizing pharmacovigilance by automating various tasks traditionally performed manually. Natural language processing (NLP) algorithms can analyze vast amounts of unstructured data from sources such as social media, electronic health records, and medical literature to detect signals of potential ADRs. These algorithms can sift through diverse sources of information, extract relevant data, and identify patterns indicative of adverse events more efficiently than human reviewers.
Moreover, ML algorithms can continuously learn from new data, improving their accuracy over time and enabling proactive identification of emerging safety concerns. By harnessing AI and ML, pharmacovigilance teams can expedite signal detection, prioritize resources effectively, and enhance their ability to respond to safety issues promptly.
2. Big Data Analytics
The proliferation of electronic health records (EHRs), claims data, and other healthcare databases has led to an abundance of healthcare data, often referred to as big data. Pharmacovigilance 2.0 leverages big data analytics to perform comprehensive safety analyses on large datasets, uncovering subtle associations and trends that may not be apparent through conventional methods.
Through advanced data mining techniques, such as cohort studies, case-control studies, and disproportionality analysis, pharmacovigilance professionals can identify potential safety signals with greater accuracy and granularity. By integrating diverse data sources and applying sophisticated analytical tools, they can gain deeper insights into drug safety profiles, facilitating more informed decision-making throughout the product lifecycle.
3. Real-World Evidence (RWE)
Real-world evidence (RWE) encompasses data collected outside the constraints of randomized controlled trials (RCTs), reflecting the everyday clinical practice and patient experiences. Pharmacovigilance 2.0 recognizes the value of RWE in complementing traditional clinical trial data by providing insights into drug safety in real-world settings.
By analyzing RWE from sources such as electronic health records, claims databases, and patient registries, pharmacovigilance professionals can evaluate the long-term safety of medications, monitor post-market outcomes, and assess the effectiveness of risk mitigation measures. RWE enables a more comprehensive understanding of drug safety and effectiveness across diverse patient populations, facilitating more personalized and evidence-based healthcare decision-making.
4. Blockchain Technology
Blockchain technology holds immense promise for enhancing the integrity, security, and transparency of pharmacovigilance data management. By utilizing a decentralized ledger system, blockchain enables secure and immutable recording of drug safety information, preventing tampering or unauthorized alterations.
In Pharmacovigilance 2.0, blockchain technology can streamline data sharing and collaboration among stakeholders while ensuring data integrity and confidentiality. Smart contracts embedded within the blockchain can automate processes such as adverse event reporting, signal detection, and regulatory compliance, reducing administrative burden and minimizing errors.
5. Predictive Analytics and Risk Stratification
Predictive analytics techniques, such as risk prediction models and decision support systems, empower pharmacovigilance professionals to proactively identify individuals at higher risk of experiencing ADRs and implement targeted interventions. By leveraging patient-specific data, including demographic factors, medical history, genetic predispositions, and concomitant medications, predictive analytics can stratify patients based on their likelihood of adverse events.
These risk stratification approaches enable personalized risk management strategies, such as tailored monitoring protocols, dose adjustments, or alternative treatment options, thereby optimizing patient safety and minimizing the burden of ADRs.
Conclusion:
Pharmacovigilance 2.0 represents a paradigm shift in drug safety monitoring, driven by the integration of next-generation technologies into safety database analysis. From artificial intelligence and big data analytics to blockchain technology and predictive analytics, these advancements are reshaping how adverse drug reactions are detected, evaluated, and managed. By harnessing the power of these technologies, pharmacovigilance professionals can enhance the efficiency, accuracy, and comprehensiveness of safety surveillance efforts, ultimately advancing public health and patient care in the era of precision medicine. As we continue to embrace innovation, collaboration, and data-driven approaches, Pharmacovigilance 2.0 holds the promise of ushering in a new era of safer and more effective medications for patients worldwide.
Comments