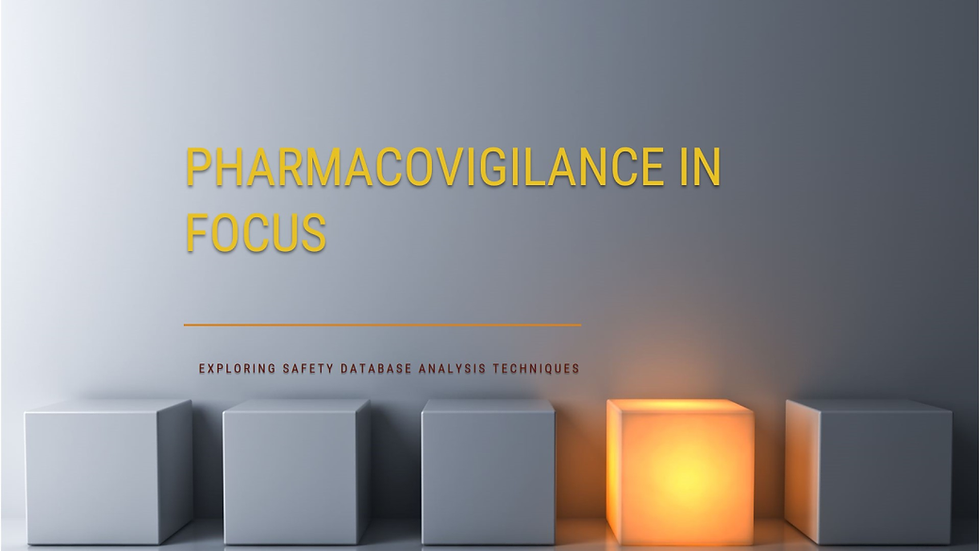
Pharmacovigilance, a cornerstone of drug safety surveillance, plays a pivotal role in monitoring and evaluating the adverse effects of pharmaceutical products post-market approval. With the increasing complexity and volume of data generated from various sources, including clinical trials and real-world evidence, effective analysis techniques are crucial for identifying potential risks and ensuring the continued safety of medications. In this article, we delve into the realm of pharmacovigilance and explore some of the key safety database analysis techniques employed in the field.
Understanding Pharmacovigilance:
Pharmacovigilance, derived from the Greek words "pharmakon" (drug) (to keep watch), encompasses the science and activities related to the detection, assessment, understanding, and prevention of adverse effects or any other drug-related problems. Its primary objective is to enhance patient safety by identifying and mitigating risks associated with pharmaceutical products throughout their lifecycle.
The Importance of Safety Database Analysis:
Safety databases serve as repositories of adverse event reports, collected from healthcare professionals, patients, and regulatory authorities worldwide. Analyzing these databases enables pharmacovigilance professionals to detect patterns, trends, and potential signals of adverse effects associated with medications. Moreover, safety database analysis plays a crucial role in signal detection, risk assessment, and regulatory decision-making.
Key Safety Database Analysis Techniques:
Descriptive Analysis: Descriptive analysis involves summarizing and characterizing the data within safety databases. This includes calculating frequencies, distributions, and proportions of adverse events reported for specific drugs or drug classes. Descriptive statistics provide an overview of the safety profile of a medication and help identify common adverse reactions.
Signal Detection: Signal detection involves the systematic identification of potential safety concerns or signals within the data. Various statistical and data mining techniques, such as disproportionality analysis and Bayesian data mining algorithms, are employed to detect statistically significant associations between drugs and adverse events. Signals identified through these analyses may warrant further investigation to assess their clinical significance.
Temporal Analysis: Temporal analysis focuses on examining the time relationship between drug exposure and the occurrence of adverse events. Methods such as the time-to-onset analysis and the proportional reporting ratio (PRR) are used to assess whether there is an increased risk of adverse events shortly after initiating treatment with a particular medication. Temporal patterns can provide insights into the potential causal relationship between drug exposure and adverse reactions.
Pattern Recognition: Pattern recognition techniques, including cluster analysis and data visualization methods, are employed to identify clusters of adverse events that may suggest previously unrecognized safety issues. By analyzing patterns of co-reported adverse events, pharmacovigilance professionals can uncover potential associations or syndromes indicative of underlying drug-related toxicity.
Risk Assessment: Risk assessment involves evaluating the magnitude and seriousness of identified safety signals to determine their clinical relevance and potential impact on public health. Quantitative risk assessment techniques, such as the calculation of risk ratios and attributable risk, aid in estimating the likelihood and severity of adverse events associated with specific drugs.
Benefit-Risk Analysis: Benefit-risk analysis integrates safety data with efficacy information to assess the overall benefit-risk profile of a medication. This involves weighing the therapeutic benefits of a drug against its potential risks and considering factors such as the severity of the underlying disease, the availability of alternative treatments, and patient preferences. Benefit-risk assessments inform regulatory decisions regarding drug approvals, labeling updates, and risk minimization strategies.
Future Directions and Challenges:
As pharmacovigilance continues to evolve, leveraging advanced data analytics, artificial intelligence, and machine learning technologies holds promise for enhancing the efficiency and effectiveness of safety database analysis. However, challenges such as data quality issues, underreporting of adverse events, and the interpretation of complex data patterns remain significant hurdles in pharmacovigilance practice.
Conclusion:
In conclusion, safety database analysis techniques are indispensable tools in pharmacovigilance for identifying, evaluating, and managing the risks associated with pharmaceutical products. By harnessing the power of data analysis, pharmacovigilance professionals can contribute to improving patient safety and public health outcomes in an increasingly complex healthcare landscape.
Comments