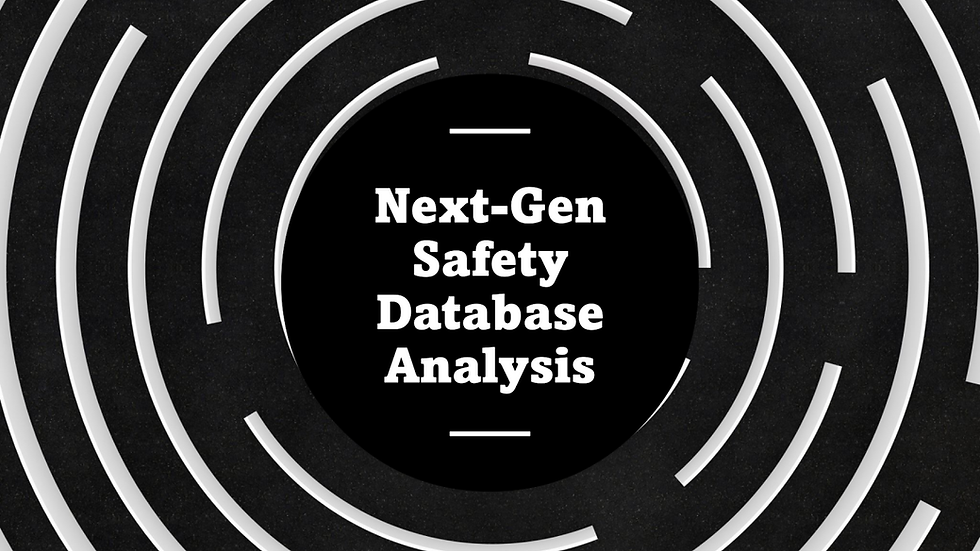
Pharmacovigilance, the science of monitoring and assessing the safety of medications, continues to evolve with advancements in technology, data analytics, and regulatory frameworks. At the heart of pharmacovigilance lies safety database analysis, where vast amounts of data are scrutinized to detect, evaluate, and mitigate potential risks associated with pharmaceutical products. In this blog, we explore the next-generation approaches to safety database analysis that are revolutionizing pharmacovigilance practices.
Harnessing Artificial Intelligence and Machine Learning:
Automated Signal Detection:
Artificial intelligence (AI) and machine learning algorithms are automating the process of signal detection in safety databases.
These algorithms analyze large volumes of data to identify patterns, associations, and signals indicative of potential adverse drug reactions, enhancing the efficiency and accuracy of pharmacovigilance surveillance.
Predictive Analytics for Risk Forecasting:
Predictive analytics models leverage machine learning techniques to forecast adverse events and predict safety outcomes.
By analyzing historical safety data and patient characteristics, predictive analytics enable proactive risk management strategies and early intervention to prevent adverse events.
Integration of Real-World Data Sources:
Incorporation of Electronic Health Records (EHRs):
Safety databases are integrating real-world data sources, such as electronic health records (EHRs), to enrich pharmacovigilance insights.
EHR data provide comprehensive information on patient demographics, medical history, and concomitant medications, enabling a deeper understanding of medication safety profiles in real-world clinical settings.
Social Media Monitoring:
Social media platforms are increasingly being utilized for pharmacovigilance surveillance, allowing for the detection of early signals and patient-reported adverse events.
Natural language processing (NLP) algorithms analyze unstructured data from social media posts, forums, and online communities to identify potential safety concerns and emerging trends related to medication use.
Advanced Data Visualization Techniques:
Interactive Dashboards and Heatmaps:
Advanced data visualization tools, such as interactive dashboards and heatmaps, enhance the accessibility and interpretability of safety database analysis results.
Stakeholders can explore and interact with data visualizations to identify trends, outliers, and clusters of adverse events, facilitating data-driven decision-making in pharmacovigilance.
Temporal Analysis and Trend Forecasting:
Temporal analysis techniques enable the visualization of temporal trends and patterns in adverse event reporting over time.
Trend forecasting models use historical data to predict future trends and anticipate shifts in medication safety profiles, guiding proactive risk management strategies and resource allocation.
Enhancing Collaboration and Data Sharing:
Global Data Harmonization Initiatives:
Collaborative efforts are underway to standardize data formats, coding systems, and terminology standards across safety databases.
Global data harmonization initiatives promote interoperability and data exchange, facilitating collaborative pharmacovigilance research and surveillance on a global scale.
Open Data Platforms and Consortia:
Open data platforms and consortia provide shared access to anonymized safety data, fostering transparency and collaboration among stakeholders.
By pooling resources and expertise, these collaborative platforms accelerate the discovery of safety signals, promote knowledge sharing, and drive innovation in pharmacovigilance practices.
Regulatory Considerations and Compliance:
Adherence to Regulatory Guidelines:
Pharmacovigilance innovations must adhere to regulatory guidelines and standards to ensure compliance with legal and ethical requirements.
Regulatory authorities provide guidance on the use of advanced analytics, real-world data sources, and AI-driven technologies in pharmacovigilance surveillance to maintain patient safety and data integrity.
Ethical Use of AI and Data Privacy:
Ethical considerations regarding the use of AI and machine learning in pharmacovigilance include transparency, accountability, and fairness in algorithmic decision-making.
Data privacy regulations, such as GDPR and HIPAA, mandate the protection of patient privacy and confidentiality in safety database analysis, requiring robust data anonymization and encryption measures.
Conclusion:
Pharmacovigilance innovations are ushering in a new era of safety database analysis, powered by artificial intelligence, real-world data integration, advanced visualization techniques, and global collaboration. By harnessing the capabilities of next-generation approaches, stakeholders can enhance the efficiency, accuracy, and timeliness of pharmacovigilance surveillance, ultimately improving patient safety and public health outcomes. However, as we embrace these innovations, it is imperative to address regulatory considerations, ethical concerns, and data privacy issues to ensure the responsible and ethical use of technology in pharmacovigilance practices. With a commitment to innovation, collaboration, and patient-centered care, pharmacovigilance professionals can leverage next-generation approaches to safeguard the well-being of patients worldwide.
Comments