Safety's Vanguard: How AI-Enabled ICSR Detection Leads the Charge
- Chaitali Gaikwad
- May 6, 2024
- 4 min read
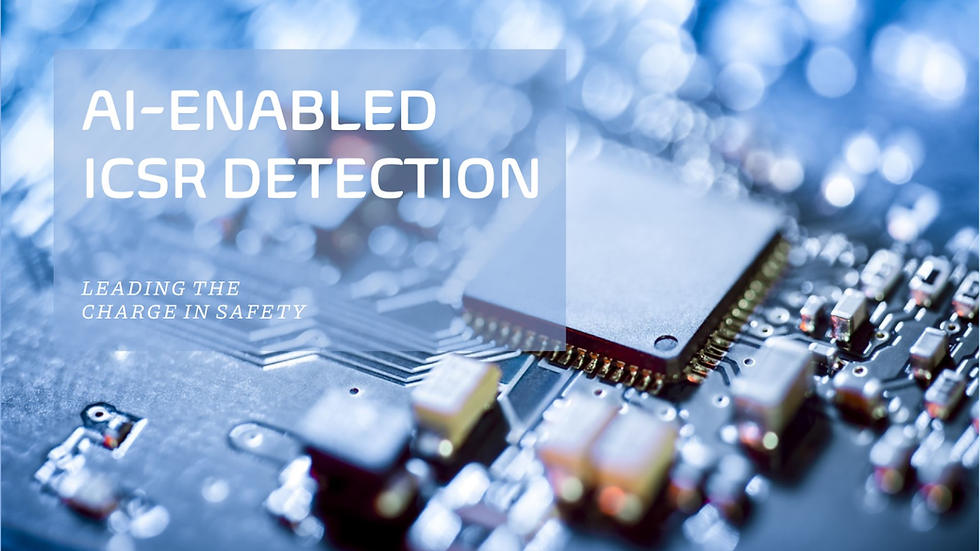
In the dynamic landscape of pharmacovigilance (PV), the pursuit of safer pharmaceuticals remains paramount. Adverse drug reactions (ADRs) pose significant risks to patient health and can have profound implications for public health systems and pharmaceutical companies alike. Identifying and reporting these adverse events promptly is crucial for mitigating harm and ensuring the continued safety of medications. In recent years, the integration of artificial intelligence (AI) technologies into PV processes has emerged as a game-changer, revolutionizing the detection and analysis of individual case safety reports (ICSRs). This blog delves into the role of AI-enabled ICSR detection as the vanguard of safety in pharmacovigilance.
Understanding the Significance of ICSR Detection:
ICSRs serve as the cornerstone of pharmacovigilance, providing real-world data on adverse events associated with pharmaceutical products. These reports, often submitted by healthcare professionals, patients, and pharmaceutical companies, contain crucial information about the nature, severity, and circumstances surrounding adverse reactions. Timely detection and assessment of ICSRs are essential for identifying potential safety concerns, facilitating regulatory action, and guiding risk management strategies.
Traditionally, the process of ICSR detection has relied heavily on manual review and analysis, which is labor-intensive, time-consuming, and prone to human error. As the volume of pharmacovigilance data continues to escalate exponentially, conventional methods struggle to keep pace with the growing influx of information. Consequently, there arises a pressing need for innovative solutions capable of augmenting human capabilities and enhancing the efficiency and accuracy of ICSR detection.
The Rise of AI in Pharmacovigilance:
Enter artificial intelligence—a disruptive force poised to revolutionize pharmacovigilance practices. AI technologies, including machine learning algorithms and natural language processing (NLP) techniques, offer unprecedented capabilities for automating and streamlining various aspects of ICSR detection and analysis. By harnessing the power of AI, pharmacovigilance stakeholders can unlock valuable insights from vast repositories of unstructured data, accelerating the identification of potential safety signals and improving decision-making processes.
Machine learning algorithms, trained on large datasets of annotated ICSRs, can learn to recognize patterns and associations indicative of adverse drug reactions. These algorithms excel at processing diverse sources of information, including free-text narratives, medical codes, and structured data fields, enabling comprehensive and context-aware analysis of safety reports. Through continuous learning and refinement, AI models can adapt to evolving pharmacovigilance challenges, enhancing their predictive accuracy and sensitivity over time.
Furthermore, NLP algorithms play a pivotal role in extracting pertinent information from unstructured text, such as narrative descriptions of adverse events. By automatically parsing and categorizing text data, NLP systems enable efficient triaging of ICSRs, prioritizing reports based on their clinical relevance and potential impact on patient safety. This intelligent screening mechanism empowers pharmacovigilance professionals to focus their attention and resources on high-priority cases, ensuring timely intervention and risk mitigation strategies.
Advantages of AI-Enabled ICSR Detection:
The integration of AI into ICSR detection offers a myriad of benefits that transcend the limitations of traditional pharmacovigilance approaches. Some of the key advantages include:
Enhanced Efficiency: AI algorithms can process vast quantities of data at unparalleled speeds, expediting the detection and analysis of ICSRs and reducing the backlog of unreviewed reports.
Improved Accuracy: By leveraging advanced analytics and pattern recognition capabilities, AI models minimize the risk of oversight and misclassification, thereby enhancing the accuracy of adverse event detection.
Early Signal Detection: AI-powered systems excel at identifying subtle safety signals and emerging trends from disparate data sources, enabling proactive risk assessment and preemptive action.
Scalability and Adaptability: AI technologies are inherently scalable and adaptable, capable of accommodating evolving data volumes and regulatory requirements without compromising performance or reliability.
Cost-Effectiveness: While initial implementation costs may be significant, AI-enabled pharmacovigilance solutions offer long-term cost savings by streamlining operational workflows and reducing manual labor expenses.
Continuous Learning: AI algorithms benefit from continuous learning and feedback loops, allowing them to iteratively improve their performance and adapt to changing patterns of adverse drug reactions.
Challenges and Considerations:
Despite its transformative potential, the adoption of AI in pharmacovigilance is not without challenges and considerations. Some of the notable issues include:
Data Quality and Bias: The effectiveness of AI models relies heavily on the quality and representativeness of training data. Biases inherent in historical data or data collection processes may inadvertently influence algorithmic outcomes, leading to skewed results or false positives/negatives.
Regulatory Compliance: Ensuring compliance with regulatory standards and guidelines poses a significant challenge in the deployment of AI-enabled pharmacovigilance systems. Stakeholders must navigate complex regulatory frameworks to validate and integrate AI technologies into existing PV workflows.
Interpretability and Transparency: The black-box nature of certain AI algorithms raises concerns regarding interpretability and transparency. Pharmacovigilance professionals require insights into the decision-making process of AI models to trust their recommendations and facilitate human oversight.
Ethical and Legal Implications: The use of AI in pharmacovigilance raises ethical and legal considerations surrounding data privacy, patient consent, and accountability. Safeguarding sensitive health information and upholding ethical standards are paramount in AI-driven PV initiatives.
Conclusion:
In conclusion, AI-enabled ICSR detection stands at the forefront of safety in pharmacovigilance, offering unprecedented capabilities for detecting, analyzing, and responding to adverse drug reactions. By harnessing the power of machine learning and natural language processing, pharmacovigilance stakeholders can unlock valuable insights from vast repositories of real-world data, enhancing the efficiency, accuracy, and timeliness of adverse event detection. While challenges remain, the transformative potential of AI in pharmacovigilance heralds a new era of proactive risk management and patient safety in the pharmaceutical industry. As we continue to navigate the complexities of drug safety monitoring, AI remains a powerful ally in our quest to safeguard public health and advance the frontiers of pharmacovigilance.
Kommentare