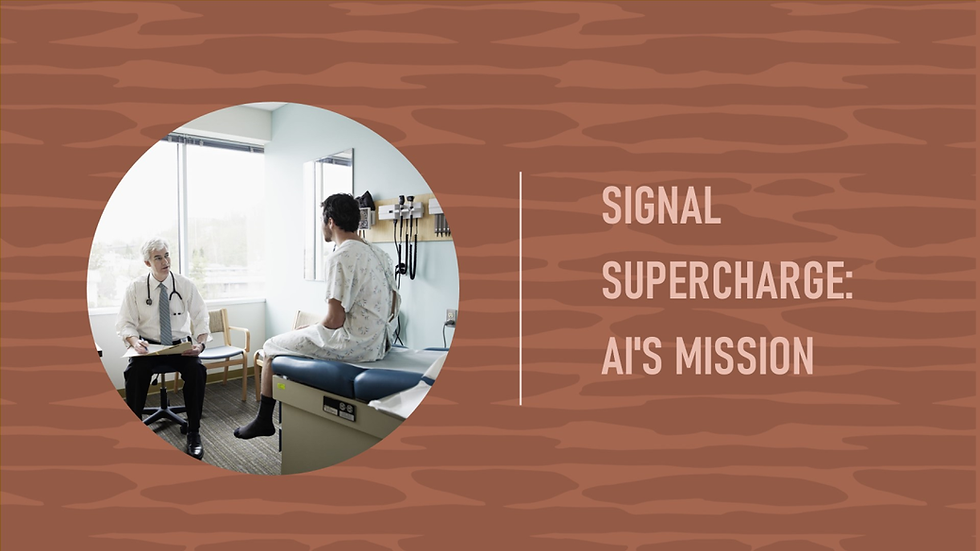
In the realm of pharmacovigilance, the ability to detect and respond to safety signals is paramount for safeguarding public health. Traditionally, signal detection has relied on manual review processes, which can be time-consuming and resource-intensive. However, with the advent of artificial intelligence (AI), there's a new frontier in signal detection—a mission to supercharge efficiency and effectiveness in drug safety monitoring. In this blog post, we'll explore how AI is revolutionizing signal detection in pharmacovigilance, amplifying efficiency and empowering healthcare professionals to stay ahead of emerging safety concerns.
Understanding Signal Detection:
Before delving into AI's role in signal detection, let's first establish a foundation by understanding what signals are and why they're important in pharmacovigilance. Signals are indications of potential safety concerns associated with medications, identified through the analysis of adverse event reports, scientific literature, and other sources of real-world data. Detecting signals early allows regulatory agencies, pharmaceutical companies, and healthcare professionals to investigate potential safety issues, take appropriate risk mitigation measures, and ensure the continued safety of medications for patients.
Challenges in Traditional Signal Detection:
Traditional methods of signal detection in pharmacovigilance often face several challenges:
Volume of Data: The sheer volume of adverse event reports and scientific literature can overwhelm manual review processes, making it difficult to identify relevant signals in a timely manner.
Complexity of Data: Adverse event reports and scientific literature contain a wealth of unstructured data, including clinical narratives, medical terminology, and patient demographics, which can be challenging to analyze using conventional methods.
Resource Constraints: Manual signal detection requires significant human resources and expertise, leading to delays in signal identification and response, particularly during periods of high reporting volume or when faced with emerging safety concerns.
AI's Role in Signal Detection:
Artificial intelligence offers a promising solution to the challenges of traditional signal detection methods. By leveraging machine learning algorithms, natural language processing (NLP) techniques, and advanced data analytics, AI systems can rapidly analyze large volumes of adverse event reports, scientific literature, social media data, and other sources of real-world evidence. These AI-powered approaches have the potential to revolutionize signal detection in pharmacovigilance by:
Automated Screening: AI algorithms can automate the screening of adverse event reports and scientific literature, rapidly identifying potential signals based on predefined criteria such as unexpectedness, seriousness, and strength of association.
Natural Language Processing: NLP techniques enable AI systems to analyze unstructured text data from adverse event reports and scientific literature, extracting key information such as adverse reactions, drug-event associations, and patient demographics.
Pattern Recognition: AI algorithms can detect patterns and trends in adverse event data, identifying clusters of similar reports or unusual temporal patterns that may indicate emerging safety concerns.
Real-time Surveillance: AI-powered surveillance systems can monitor adverse event reports and other sources of real-world data in real time, providing early warning of potential safety signals and enabling proactive risk mitigation measures.
Case Studies in AI-Powered Signal Detection:
To illustrate the impact of AI on signal detection in pharmacovigilance, let's consider a few hypothetical case studies:
Cluster Analysis: An AI algorithm analyzes a large database of adverse event reports and identifies a cluster of reports describing a rare adverse reaction associated with a newly approved medication. Regulatory agencies and pharmaceutical companies investigate further, leading to a safety communication and label update.
Literature Mining: An AI-powered literature mining tool scans scientific journals and identifies a series of case reports describing a previously unrecognized drug-drug interaction. Healthcare professionals are alerted to the potential risk, leading to changes in prescribing practices and improved patient safety.
Social Media Monitoring: An AI system monitors social media platforms for mentions of adverse drug reactions and detects a sudden increase in reports of a known side effect associated with a widely used medication. Healthcare providers are alerted to the trend, prompting closer monitoring of patients and additional risk communication efforts.
Benefits and Challenges of AI-Powered Signal Detection:
While AI holds tremendous promise for supercharging efficiency in drug safety monitoring, it's important to acknowledge both the benefits and challenges:
Benefits:
Speed and Efficiency: AI-powered signal detection can analyze large volumes of data rapidly, enabling earlier detection of safety signals and faster response times.
Accuracy and Consistency: AI algorithms can standardize signal detection criteria and minimize human bias, leading to more consistent and reliable results.
Scalability: AI systems can scale to handle increasing volumes of adverse event reports and other sources of real-world data, providing a scalable solution for pharmacovigilance activities.
Challenges:
Data Quality: AI algorithms are only as good as the data they're trained on, and issues such as data quality, completeness, and bias can impact the accuracy of signal detection results.
Interpretability: The black-box nature of some AI algorithms may make it difficult to interpret how decisions are made, raising concerns about transparency and accountability in signal detection.
Regulatory Acceptance: Regulatory agencies may require evidence of the reliability and performance of AI algorithms before incorporating them into pharmacovigilance processes, posing challenges for widespread adoption.
Conclusion:
In conclusion, AI is on a mission to supercharge efficiency in drug safety monitoring by revolutionizing signal detection in pharmacovigilance. By automating screening, leveraging NLP techniques, and analyzing large volumes of real-world data, AI-powered systems have the potential to identify safety signals earlier, enabling proactive risk mitigation measures and ensuring the continued safety of medications for patients worldwide. While challenges remain, ongoing research and innovation in the field of AI hold the promise of further advancements in signal detection methods, ultimately benefiting patients, healthcare providers, and society as a whole. As we continue to harness the power of AI in pharmacovigilance, the mission to amplify efficiency in drug safety through signal supercharge continues to unfold.
Comentarios