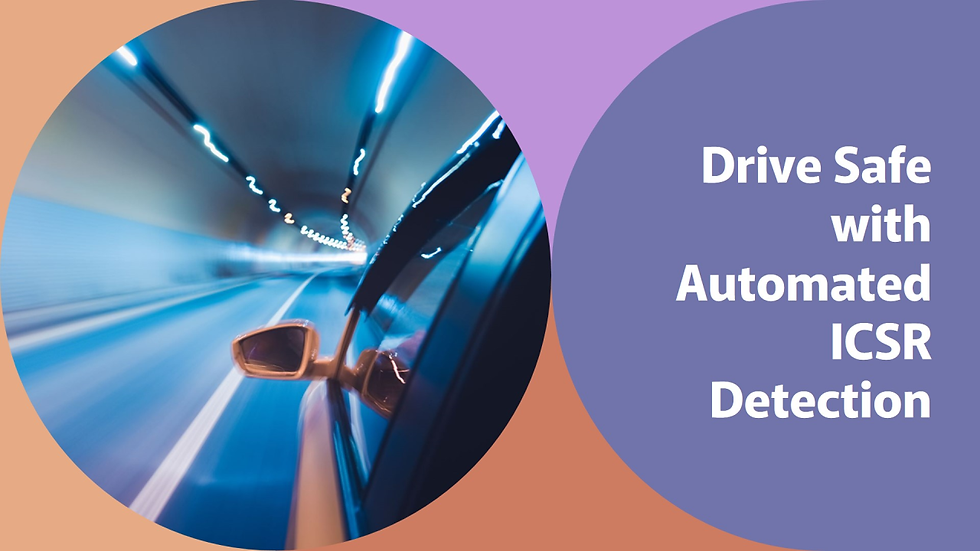
In the realm of pharmacovigilance, the timely detection and analysis of Individual Case Safety Reports (ICSRs) are critical for ensuring the safety of medicinal products. However, the manual processing of ICSRs can be time-consuming and resource-intensive, leading to delays in signal detection and risk assessment. Automated ICSR detection technologies offer a solution to this challenge by leveraging advanced algorithms and machine learning techniques to streamline the identification and analysis of safety data. In this blog, we will explore the benefits, challenges, and best practices associated with automated ICSR detection, highlighting its potential to accelerate safety analysis and improve patient outcomes.
Understanding Individual Case Safety Reports (ICSRs):
Individual Case Safety Reports (ICSRs) are reports of suspected adverse reactions or other drug-related problems associated with the use of medicinal products. ICSRs contain detailed information about the patient, the suspected medication, the adverse event, and any relevant medical history or concomitant medications. Pharmacovigilance professionals analyze ICSRs to identify potential safety signals, assess the risk-benefit profile of medicinal products, and take appropriate regulatory actions to protect public health.
Challenges with Manual ICSR Detection:
Manual ICSR detection involves the labor-intensive process of reviewing and analyzing large volumes of safety data collected from various sources, including healthcare professionals, patients, and regulatory authorities. This process can be slow, error-prone, and resource-intensive, leading to delays in signal detection and risk assessment. Additionally, manual ICSR detection may result in inconsistencies and variations in data interpretation, impacting the quality and reliability of safety analysis.
Benefits of Automated ICSR Detection:
Automated ICSR detection technologies offer several advantages over manual processes, including speed, accuracy, and scalability. By leveraging advanced algorithms and machine learning techniques, automated systems can rapidly process and analyze large volumes of safety data, enabling pharmacovigilance professionals to identify potential safety signals more quickly and efficiently. Automated ICSR detection also reduces the risk of human error and variability, leading to more consistent and reliable safety analysis.
Key Components of Automated ICSR Detection Systems:
Automated ICSR detection systems typically consist of several key components, including data collection, preprocessing, signal detection, and validation. Data collection involves gathering ICSRs from various sources, such as electronic health records, spontaneous reporting systems, and social media platforms. Preprocessing techniques are then applied to clean and standardize the data, ensuring consistency and accuracy. Signal detection algorithms analyze the processed data to identify potential safety signals, which are then validated through further investigation and analysis.
Challenges and Considerations:
While automated ICSR detection offers significant benefits, several challenges and considerations must be addressed to ensure its successful implementation. These include data quality and completeness, algorithm validation and performance assessment, regulatory compliance, and integration with existing pharmacovigilance systems and workflows. Additionally, ethical and privacy considerations must be carefully managed when collecting and analyzing sensitive patient data.
Best Practices for Implementing Automated ICSR Detection:
To maximize the benefits of automated ICSR detection, pharmaceutical companies and regulatory authorities should adopt best practices for implementation and validation. This includes establishing robust data governance processes to ensure data quality and integrity, validating algorithms against gold standard datasets, conducting regular performance assessments, and maintaining transparency and accountability throughout the process. Collaboration and knowledge sharing among stakeholders are also essential for advancing the field of automated pharmacovigilance.
Conclusion:
Automated ICSR detection holds tremendous promise for accelerating safety analysis and improving patient outcomes in pharmacovigilance. By leveraging advanced algorithms and machine learning techniques, automated systems can streamline the identification and analysis of safety data, enabling pharmacovigilance professionals to detect potential safety signals more quickly and efficiently. While challenges and considerations exist, proactive measures and best practices can help overcome these obstacles and unlock the full potential of automated ICSR detection in enhancing drug safety and public health.
Comments