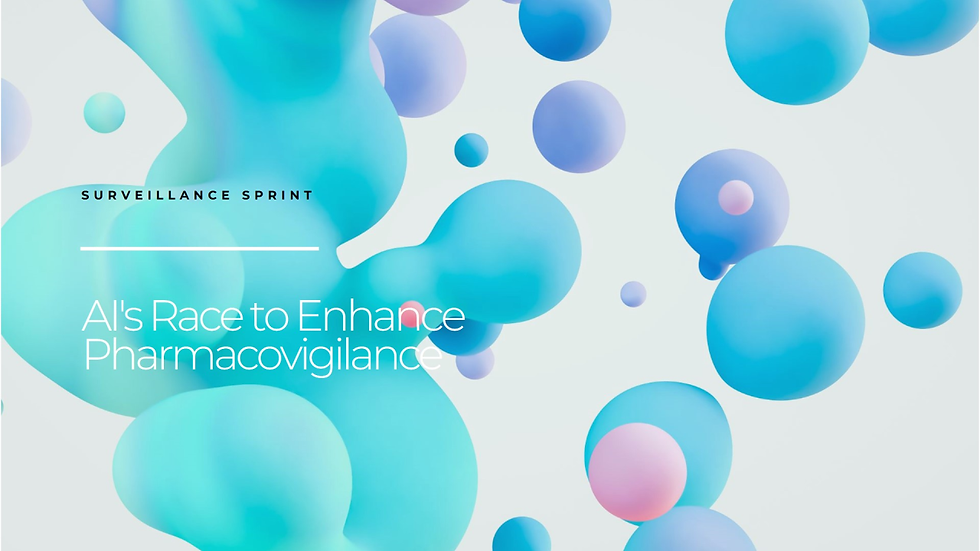
In the intricate world of pharmaceuticals, ensuring patient safety is paramount. The process of detecting, assessing, understanding, and preventing adverse effects or any other drug-related problems is known as pharmacovigilance. As drug development progresses and the volume of data increases, the need for efficient, accurate, and timely pharmacovigilance systems has never been greater. Enter artificial intelligence (AI), a game-changer poised to revolutionize the field.
The Current Landscape of Pharmacovigilance:
Pharmacovigilance (PV) is a critical component of healthcare that focuses on the safety of drugs and medical devices. Traditional PV processes involve the collection and analysis of adverse event reports, clinical trial data, and real-world evidence. These tasks are often labor-intensive, time-consuming, and prone to human error.
The primary goal of PV is to identify adverse drug reactions (ADRs) and ensure they are reported and addressed promptly. This is vital not only for patient safety but also for regulatory compliance. Pharmaceutical companies must adhere to strict guidelines set by regulatory bodies such as the FDA (Food and Drug Administration) in the United States and the EMA (European Medicines Agency) in Europe.
Challenges in Traditional Pharmacovigilance:
Volume and Complexity of Data: The sheer volume of data generated from clinical trials, electronic health records (EHRs), social media, and other sources can be overwhelming. Traditional methods struggle to keep up with the influx of information.
Delayed Reporting: Manual processes can lead to delays in reporting adverse events. This lag can have serious implications for patient safety.
Inconsistent Data Quality: The quality of data can vary significantly, with discrepancies in how adverse events are reported and documented. This inconsistency can hinder accurate analysis.
Resource Intensive: The traditional PV process is labor-intensive, requiring significant human resources to manually review and analyze data.
Global Regulatory Requirements: Different countries have varying regulations and requirements for pharmacovigilance, adding a layer of complexity to the process.
Case Studies: AI in Action
Several pharmaceutical companies and regulatory agencies are already leveraging AI to enhance their pharmacovigilance efforts. Here are a few notable examples:
IBM Watson, a leading AI platform, has been utilized in various pharmacovigilance applications. Watson’s NLP capabilities allow it to analyze unstructured data from medical literature, clinical trial reports, and social media. By identifying potential safety signals, Watson helps pharmaceutical companies to detect and address ADRs more efficiently.
AstraZeneca, a global biopharmaceutical company, has implemented an AI-driven pharmacovigilance system to streamline adverse event reporting and analysis. The system uses machine learning algorithms to process adverse event data, identify potential safety signals, and generate regulatory reports. This has significantly reduced the time and effort required for PV activities.
The FDA’s Sentinel Initiative is a national electronic system designed to monitor the safety of FDA-regulated medical products. Leveraging AI and big data analytics, the Sentinel Initiative analyzes data from EHRs, insurance claims, and registries to detect safety signals. This proactive approach enables the FDA to identify and respond to potential safety concerns more rapidly.
Challenges and Considerations:
While AI offers significant benefits for pharmacovigilance, its implementation is not without challenges. Here are a few considerations:
AI systems rely on high-quality data for accurate analysis. Ensuring the integrity and consistency of data from diverse sources is crucial for the effectiveness of AI-driven pharmacovigilance.
Regulatory bodies must recognize and accept AI-driven approaches to pharmacovigilance. This requires collaboration between pharmaceutical companies, technology providers, and regulators to establish guidelines and standards for AI use in PV.
The use of AI in pharmacovigilance raises ethical and privacy concerns, particularly regarding the handling of sensitive patient data. It is essential to implement robust data protection measures and ensure compliance with regulations such as GDPR (General Data Protection Regulation).
Integrating AI solutions with existing pharmacovigilance systems and workflows can be challenging. Companies must invest in the necessary infrastructure and training to ensure seamless integration and maximize the benefits of AI.
The Future of Pharmacovigilance
The integration of AI into pharmacovigilance represents a significant advancement in the field. As AI technologies continue to evolve, their capabilities will expand, offering even greater efficiencies and insights. Here are a few trends to watch:
1. Real-Time Pharmacovigilance
Advancements in AI and big data analytics will enable real-time pharmacovigilance, where adverse events are detected and addressed as they occur. This proactive approach will enhance patient safety and reduce the time to market for new drugs.
2. Personalized Medicine
AI will play a crucial role in the development of personalized medicine, where treatments are tailored to individual patients based on their genetic makeup and other factors. In pharmacovigilance, this will involve identifying ADRs specific to certain patient populations and developing targeted mitigation strategies.
3. Collaboration and Data Sharing
The future of pharmacovigilance will involve greater collaboration and data sharing between pharmaceutical companies, healthcare providers, and regulatory bodies. AI will facilitate this by standardizing data and providing a common platform for analysis and reporting.
4. Continuous Learning and Improvement
AI systems will continuously learn and improve from new data and experiences. This iterative process will enhance the accuracy and effectiveness of pharmacovigilance activities, ensuring that patient safety remains a top priority.
Conclusion:
AI is poised to revolutionize pharmacovigilance by enhancing the efficiency, accuracy, and speed of adverse event detection and analysis. By leveraging machine learning, natural language processing, and predictive analytics, AI can address the challenges of traditional PV methods and ensure that patient safety is maintained in an increasingly complex healthcare landscape.
Comments