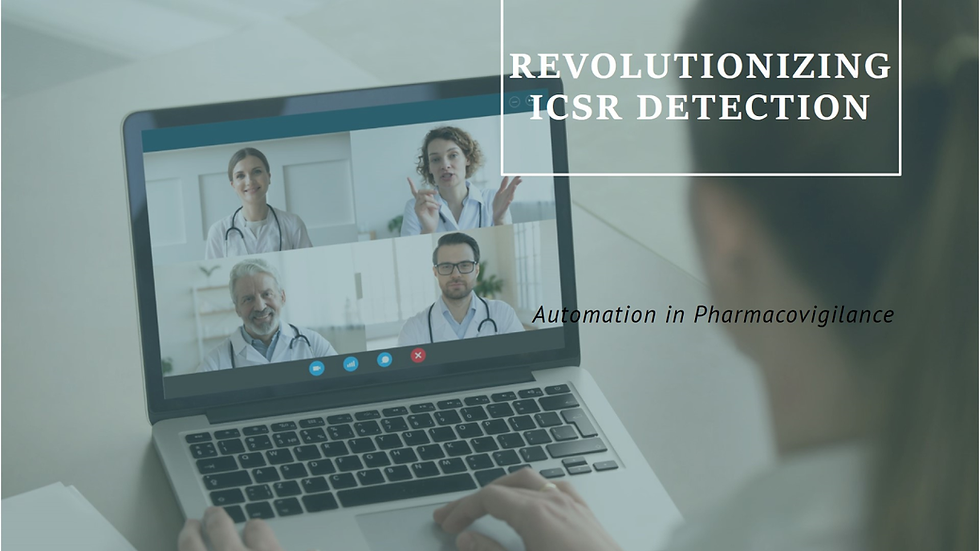
In the fast-paced world of pharmacovigilance, where monitoring adverse drug reactions is critical to patient safety, automation is emerging as a game-changer. Traditionally, Individual Case Safety Report (ICSR) detection relied heavily on manual processes, making it time-consuming and prone to errors.
However, with advancements in technology, particularly in natural language processing (NLP) and machine learning, the landscape of pharmacovigilance is undergoing a transformation. This blog explores how automation is revolutionizing ICSR detection, streamlining processes, and enhancing patient safety.
The Challenge of Manual ICSR Detection:
Manual ICSR detection involves sifting through vast amounts of unstructured data from diverse sources such as medical literature, regulatory reports, and adverse event databases. Human analysts must review each report to identify potential adverse drug reactions, a process that is labor-intensive, time-consuming, and susceptible to human error. Furthermore, the sheer volume of data generated daily poses a significant challenge, overwhelming traditional manual surveillance systems.
The Rise of Automation:
Automation, fueled by advances in AI and NLP, offers a solution to the limitations of manual ICSR detection. Machine learning algorithms can be trained on large datasets of labeled ICSRs to recognize patterns and identify potential adverse events automatically. NLP techniques enable the extraction of relevant information from unstructured text sources, such as clinical notes and social media posts, enriching the data available for analysis. By automating ICSR detection, organizations can significantly increase efficiency, reduce workload, and improve the timeliness and accuracy of adverse event reporting.
Applications of Automation in Pharmacovigilance:
Automation is revolutionizing various aspects of pharmacovigilance beyond ICSR detection. Machine learning algorithms can prioritize ICSRs based on factors such as severity, likelihood, and patient demographics, enabling faster and more targeted responses to potential safety concerns. Furthermore, automation can facilitate signal detection by analyzing trends and patterns in adverse event data, helping identify emerging risks early.
Another promising application of automation is the integration of real-world data sources, such as electronic health records (EHRs) and wearable devices, into pharmacovigilance systems. By leveraging these rich sources of patient data, organizations can enhance their understanding of drug safety profiles and detect adverse events more comprehensively.
Challenges and Considerations:
Despite its potential benefits, automation in pharmacovigilance also presents challenges and considerations. Data quality and standardization remain critical issues, as automated algorithms rely on accurate and reliable data inputs for optimal performance. Furthermore, ensuring the transparency and interpretability of AI models is essential for maintaining trust and confidence in automated surveillance systems. Ethical considerations, such as patient privacy and consent, also need to be carefully addressed to uphold ethical standards in pharmacovigilance practices.
Future Directions:
The future of pharmacovigilance lies at the intersection of automation, data analytics, and collaboration. As technology continues to evolve, so too will the capabilities of automated surveillance systems. Integrating AI-driven automation with other emerging technologies, such as blockchain and real-world evidence, holds promise for further enhancing the efficiency and effectiveness of pharmacovigilance efforts.
Furthermore, fostering collaboration between stakeholders, including regulatory agencies, healthcare providers, pharmaceutical companies, and technology vendors, is crucial for driving innovation and advancing the field of pharmacovigilance. By working together, we can harness the power of automation to ensure the safety and well-being of patients worldwide.
Conclusion:
Automation is revolutionizing ICSR detection in pharmacovigilance, transforming how adverse drug reactions are monitored and managed. By leveraging AI and NLP technologies, organizations can streamline processes, enhance data analysis capabilities, and improve patient safety outcomes. While challenges and considerations remain, the potential benefits of automation in pharmacovigilance are profound. As we embrace this technological revolution, we must continue to prioritize data quality, transparency, and ethical standards to realize the full potential of automation in safeguarding public health.
Comments