The Evolving Landscape of Pharmacovigilance: Trends in Safety Database Utilization
- Chaitali Gaikwad
- Apr 22, 2024
- 3 min read
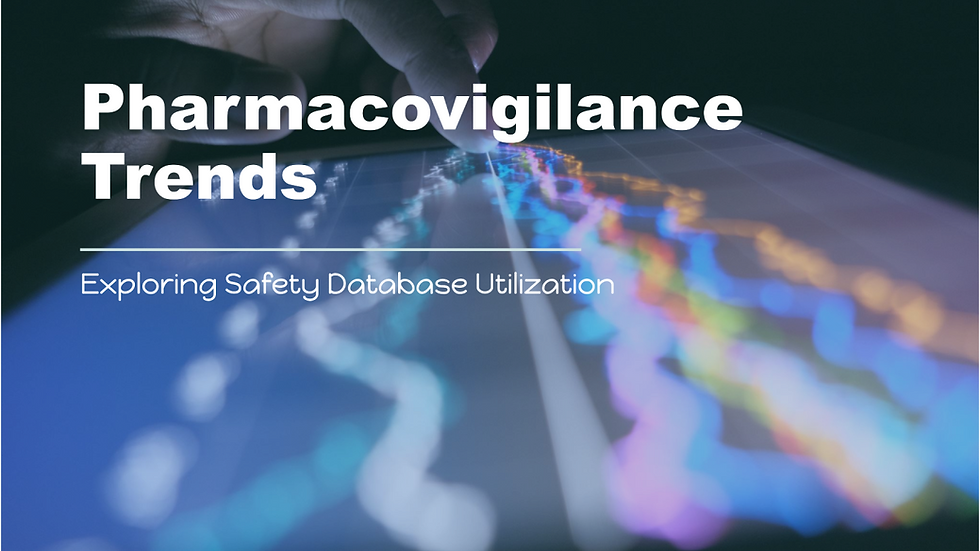
In the realm of healthcare, pharmacovigilance stands as a sentinel, safeguarding public health by monitoring the safety of medications. Over the years, advancements in technology, regulatory frameworks, and healthcare practices have transformed the landscape of pharmacovigilance. Central to this evolution is the utilization of safety databases, which serve as invaluable repositories of information on adverse drug reactions and other safety-related data. This blog explores the emerging trends and innovations shaping the utilization of safety databases in pharmacovigilance.
Harnessing Real-World Data:
Integration of Real-World Evidence (RWE):
Pharmaceutical companies and regulatory agencies are increasingly leveraging real-world data sources, such as electronic health records (EHRs), claims databases, and social media platforms, to supplement traditional clinical trial data.
Safety databases are evolving to incorporate RWE, providing insights into medication use patterns, treatment outcomes, and patient experiences in real-world settings.
Utilization of Wearable Devices and IoT:
The proliferation of wearable devices and Internet of Things (IoT) technology offers new avenues for collecting real-time health data.
Safety databases are integrating data from wearable devices, such as fitness trackers and smartwatches, to monitor medication adherence, detect adverse events, and assess patient responses to treatment.
Enhancing Data Analytics Capabilities:
Adoption of Big Data Analytics:
The exponential growth in healthcare data necessitates advanced analytics techniques to derive meaningful insights.
Safety databases are leveraging big data analytics tools and machine learning algorithms to identify patterns, trends, and signals indicative of potential safety issues.
Predictive Analytics for Early Signal Detection:
Predictive analytics models are being employed to forecast adverse events and identify emerging safety signals before they escalate.
By analyzing historical safety data and risk factors, predictive analytics enable proactive risk management and intervention strategies.
Improving Signal Detection and Risk Assessment:
Signal Detection Algorithms:
Advanced signal detection algorithms are enhancing the efficiency and accuracy of adverse event detection.
These algorithms utilize data mining techniques, statistical analyses, and machine learning algorithms to identify potential safety signals from large-scale datasets.
Benefit-Risk Assessment Frameworks:
Pharmacovigilance practitioners are adopting comprehensive benefit-risk assessment frameworks to evaluate the safety profiles of medications.
These frameworks integrate safety database findings with clinical trial data, patient preferences, and healthcare outcomes to make informed decisions about drug safety and regulatory actions.
Promoting Collaboration and Data Sharing:
Global Data Sharing Initiatives:
International collaborations, such as the WHO Programming for International Drug Monitoring and the FDA's Sentinel Initiative, facilitate data sharing and surveillance on a global scale.
Safety databases serve as platforms for sharing anonymized safety data, promoting transparency, and fostering collaborative research efforts.
Interoperability and Standardization:
Efforts to standardize data formats, coding systems, and terminologies enhance interoperability and data exchange among safety databases.
Adoption of common standards, such as MedDRA and SNOMED CT, promotes consistency and facilitates data integration across diverse data sources.
Addressing Privacy and Ethical Considerations:
Privacy-Preserving Data Sharing:
Privacy-preserving techniques, such as data anonymization and encryption, safeguard patient privacy while enabling data sharing for research purposes.
Compliance with regulatory requirements, such as HIPAA and GDPR, ensures the ethical and responsible use of healthcare data.
Ethical Use of AI and Predictive Analytics:
Ethical guidelines and frameworks govern the use of artificial intelligence and predictive analytics in pharmacovigilance.
Transparency, accountability, and fairness are paramount in the development and deployment of AI-driven safety database solutions.
Conclusion:
The utilization of safety databases in pharmacovigilance is undergoing a paradigm shift, driven by technological innovation, data analytics advancements, and collaborative initiatives. From harnessing real-world data to enhancing data analytics capabilities and promoting global collaboration, safety databases are evolving to meet the dynamic challenges of drug safety surveillance. By embracing emerging trends and leveraging the power of data-driven insights, stakeholders can navigate the evolving landscape of pharmacovigilance and advance the mission of ensuring the safe and effective use of medications for all.
Comments