The Role of Artificial Intelligence in Pharmacovigilance and Safety Databases
- Chaitali Gaikwad
- Apr 20, 2024
- 3 min read
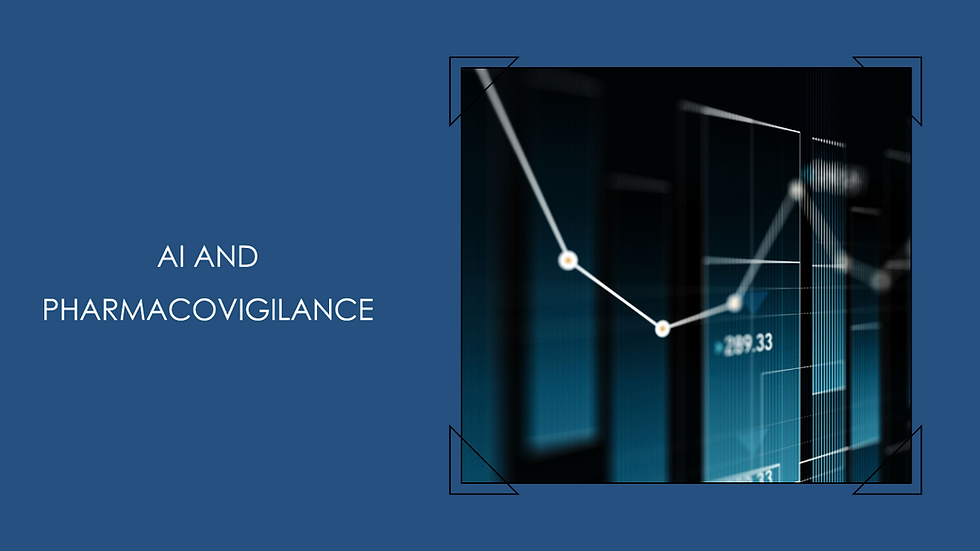
In the ever-evolving landscape of healthcare, where advancements are ceaselessly pushing the boundaries of innovation, one domain stands out for its criticality: pharmacovigilance. Ensuring the safety of pharmaceutical products is paramount, and the advent of artificial intelligence (AI) has revolutionized the methodologies employed in this crucial field. From streamlining data collection to enhancing signal detection, AI is reshaping pharmacovigilance and safety databases in ways previously unimaginable.
Understanding Pharmacovigilance
Pharmacovigilance, often referred to as drug safety surveillance, is the science and activities related to the detection, assessment, understanding, and prevention of adverse effects or any other drug-related problems. It encompasses a broad spectrum of tasks, including the collection of adverse event reports, signal detection for potential safety concerns, risk management, and regulatory compliance.
The Need for AI in Pharmacovigilance
Traditional pharmacovigilance methods have long relied on manual processes, making them susceptible to human error, inefficiencies, and the inability to handle the vast amounts of data generated. With the exponential growth in healthcare data, there arises a pressing need for advanced technologies to extract meaningful insights and ensure timely interventions.
AI presents a compelling solution to these challenges. By leveraging machine learning algorithms, natural language processing (NLP), and other AI techniques, pharmacovigilance processes can be automated, accelerated, and made more accurate.
Let's delve into the multifaceted role of AI in pharmacovigilance and safety databases:
1. Data Collection and Processing
One of the primary tasks in pharmacovigilance is the collection and processing of adverse event reports from various sources, including healthcare professionals, patients, and regulatory authorities. AI-powered systems can automate this process by extracting relevant information from unstructured data sources such as electronic health records, social media, and medical literature. NLP algorithms enable the parsing and categorization of text, facilitating the identification of adverse events and their associated medications.
2. Signal Detection and Analysis
Identifying potential safety signals amidst vast datasets is a daunting task for human analysts. AI algorithms excel in this domain by continuously analyzing data patterns to detect unusual correlations or clusters of adverse events that may indicate previously unknown safety concerns. Machine learning models can learn from historical data to prioritize signals for further investigation, thereby enhancing the efficiency of pharmacovigilance activities.
3. Predictive Analytics for Risk Assessment
AI-powered predictive analytics offer a proactive approach to risk assessment in pharmacovigilance. By analyzing patient data, drug characteristics, and other relevant factors, predictive models can forecast the likelihood of adverse events occurring in specific patient populations. This enables healthcare providers and regulatory agencies to take preemptive measures, such as implementing risk mitigation strategies or issuing safety warnings, to protect patient safety.
4. Drug Interaction and Adverse Event Prediction
AI algorithms can analyze complex interactions between drugs, diseases, and patient demographics to predict potential adverse events or drug-drug interactions. By integrating diverse datasets and utilizing advanced analytical techniques, AI systems can identify subtle relationships that may not be apparent through traditional methods. This predictive capability empowers healthcare professionals to make informed decisions regarding drug therapy and patient management.
5. Real-time Monitoring and Surveillance
In the era of big data, real-time monitoring of drug safety is essential to detect emerging risks and respond promptly. AI-driven surveillance systems continuously monitor healthcare databases, social media platforms, and other sources for signals of adverse events or medication errors. By leveraging advanced algorithms and automation, these systems enable rapid signal detection and facilitate timely interventions to mitigate risks to patient safety.
Challenges and Future Directions
While the integration of AI in pharmacovigilance holds immense promise, it is not without challenges. Ensuring the accuracy, reliability, and interpretability of AI algorithms remains a critical concern. Moreover, regulatory frameworks must evolve to accommodate the use of AI-driven technologies in drug safety surveillance.
Looking ahead, the future of pharmacovigilance lies in the convergence of AI with other cutting-edge technologies such as blockchain and the Internet of Things (IoT). Blockchain technology can enhance data integrity and traceability, while IoT devices enable real-time monitoring of patient outcomes and medication adherence.
Conclusion:
The role of artificial intelligence in pharmacovigilance and safety databases is transformative, offering unprecedented opportunities to enhance drug safety surveillance and protect public health. By automating labor-intensive tasks, accelerating signal detection, and enabling predictive analytics, AI empowers healthcare stakeholders to make data-driven decisions and ensure the timely detection and management of adverse events. As AI continues to evolve, its integration into pharmacovigilance processes will shape the future of drug safety monitoring, ultimately benefiting patients worldwide.
Comentários