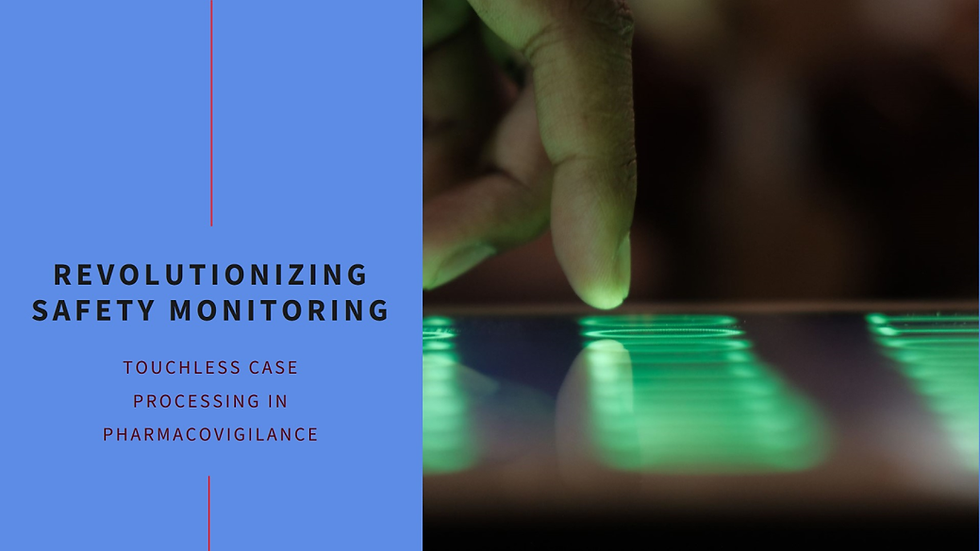
In the fast-paced world of pharmacovigilance, where the safety and efficacy of medicinal products are paramount, the need for efficient and accurate case processing is ever-present. Traditionally, case processing involved numerous manual steps, often prone to errors and delays. However, with advancements in technology, particularly in artificial intelligence (AI) and automation, a new era of touchless case processing has emerged, promising to revolutionize the field.
Traditional Case Processing vs. Touchless Case Processing:
In the traditional approach to case processing, healthcare professionals manually collect and input data from disparate sources, such as spontaneous reports, literature databases, and electronic health records. This process is not only time-consuming but also susceptible to human error and inconsistencies. Conversely, touchless case processing automates various stages of the workflow, from data ingestion to assessment and reporting, minimizing human intervention and maximizing efficiency.
Touchless case processing refers to the automation of various stages in the pharmacovigilance workflow, from case intake to assessment and reporting, with minimal human intervention. This innovative approach harnesses the power of AI algorithms, natural language processing (NLP), machine learning (ML), and robotic process automation (RPA) to streamline processes and enhance efficiency while maintaining the highest standards of safety monitoring.
Impactful Innovations in Touchless Case Processing:
Case Intake and Triage:
Automation of data ingestion from diverse sources such as spontaneous reports, literature databases, electronic health records, and social media platforms.
Application of NLP algorithms to extract relevant information from unstructured data sources, including medical narratives and scientific articles.
Automatic triaging of cases based on predefined criteria, such as seriousness, expectedness, and regulatory reporting requirements.
Data Standardization and Coding:
Automated coding of adverse events and medical terms using standardized dictionaries such as MedDRA (Medical Dictionary for Regulatory Activities) and WHO Drug.
Integration of coding algorithms with case management systems to ensure consistency and accuracy in data capture.
Signal Detection and Prioritization:
Utilization of ML algorithms to analyze pharmacovigilance data and identify potential safety signals.
Automated generation of signal detection algorithms based on historical data and statistical methodologies.
Prioritization of signals based on predefined criteria, such as severity, frequency, and clinical relevance.
Case Assessment and Causality Analysis:
Automation of case assessment processes, including causality assessment and severity grading.
Integration of AI-based decision support tools to assist pharmacovigilance professionals in evaluating the likelihood of a causal relationship between a drug and an adverse event.
Automated generation of case narratives and summary reports for regulatory submissions.
Regulatory Reporting and Compliance:
Automatic generation of regulatory reports, including Individual Case Safety Reports (ICSRs) and Periodic Safety Update Reports (PSURs), in compliance with global regulatory requirements.
Integration of regulatory intelligence tools to ensure adherence to evolving pharmacovigilance regulations and guidelines.
How Touchless Case Processing is Possible:
Datacreds's Salvus - Drug Safety database and Ingest - Data Ingestion system are prime examples of how touchless case processing is achieved in pharmacovigilance.
Salvus - Drug Safety Database:
Salvus is a state-of-the-art pharmacovigilance database developed by Datacreds, designed to automate and streamline case processing workflows.
Leveraging advanced AI and ML algorithms, Salvus automates data ingestion from diverse sources, including spontaneous reports, electronic health records, and scientific literature databases.
The platform employs NLP techniques to extract relevant information from unstructured data sources, such as medical narratives and social media posts, enabling comprehensive safety monitoring.
Ingest - Data Ingestion System:
Ingest is an innovative data ingestion system developed by Datacreds, designed to seamlessly integrate with Salvus and automate the ingestion of pharmacovigilance data.
Ingest employs RPA technologies to automate the extraction and ingestion of data from disparate sources, eliminating the need for manual data entry and reducing the risk of errors and inconsistencies.
The system utilizes advanced data standardization algorithms to ensure consistency and accuracy in data capture, facilitating downstream processes such as signal detection and regulatory reporting.
By combining Salvus - Drug Safety database with Ingest - Data Ingestion system, Datacreds has achieved touchless case processing in pharmacovigilance, enabling organizations to automate and streamline case processing workflows while maintaining the highest standards of safety monitoring and regulatory compliance. Through the integration of AI, NLP, ML, and RPA technologies, Datacreds has paved the way for the future of pharmacovigilance, empowering organizations to enhance efficiency, accuracy, and scalability in safety monitoring practices.
Ready to see it in action? Request a demo today.
Comments