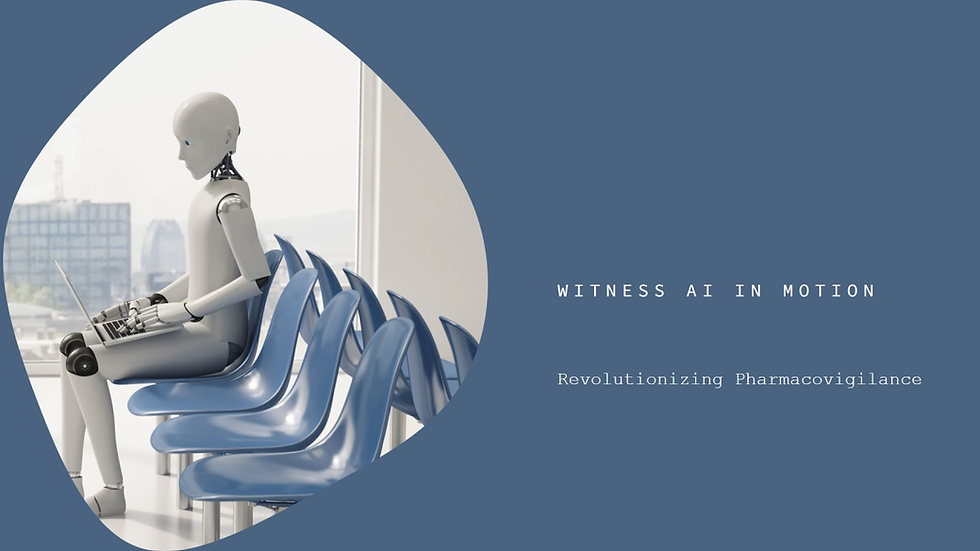
In the fast-paced world of pharmacovigilance, the integration of Artificial Intelligence (AI) is propelling a seismic shift in how we interact with pharmacovigilance literature. This blog embarks on an exploration of the transformative power of AI in pharmacovigilance literature, uncovering its impact on data analysis, signal detection, and the future of drug safety monitoring.
The Role of Pharmacovigilance Literature:
Pharmacovigilance literature serves as the cornerstone of drug safety surveillance, housing a wealth of information on adverse drug reactions (ADRs), medication errors, and post-market surveillance data. From clinical trials to real-world evidence, pharmacovigilance literature provides insights crucial for assessing drug safety, identifying emerging risks, and informing regulatory decisions.
The Emergence of AI in Pharmacovigilance:
The advent of AI technologies, including machine learning, natural language processing (NLP), and data analytics, has catalyzed a paradigm shift in pharmacovigilance literature analysis. AI algorithms are capable of parsing vast volumes of unstructured data, extracting meaningful insights, and detecting subtle patterns that may elude human analysts. This capability has unlocked new possibilities for enhancing the efficiency, accuracy, and timeliness of pharmacovigilance activities.
Revolutionizing Data Analysis:
AI-driven data analysis tools are revolutionizing the way pharmacovigilance literature is analyzed:
Automated Literature Screening: AI algorithms can sift through extensive databases of scientific literature, identifying relevant studies, and prioritizing articles for review based on predefined criteria. This automated screening process accelerates literature review timelines and minimizes the risk of oversight.
Semantic Search and Entity Recognition: NLP techniques enable AI systems to perform semantic searches and extract key entities, such as drug names, adverse events, and patient demographics, from unstructured text. This semantic understanding enhances the precision and relevance of literature analysis, enabling researchers to pinpoint relevant information quickly.
Topic Modeling and Clustering: AI-powered topic modeling algorithms can categorize pharmacovigilance literature into thematic clusters, uncovering hidden relationships and trends within the data. This clustering facilitates knowledge discovery, hypothesis generation, and the identification of novel insights that may inform future research directions.
Empowering Signal Detection:
Signal detection lies at the heart of pharmacovigilance, and AI is revolutionizing this critical process:
Predictive Analytics: AI models leverage historical pharmacovigilance data to predict the likelihood of adverse events associated with specific drugs or patient populations. These predictive models enable proactive risk management strategies and early intervention to mitigate potential safety concerns.
Anomaly Detection: AI algorithms can flag unusual patterns or deviations in pharmacovigilance data, signaling potential safety signals that merit further investigation. By detecting outliers and anomalies in real-time, AI enhances the sensitivity and specificity of signal detection algorithms, reducing false positives and negatives.
Adverse Event Prediction: AI-driven predictive analytics can forecast the probability of adverse events occurring in patient populations based on drug exposure, patient characteristics, and other contextual factors. This proactive approach enables healthcare providers to anticipate and prevent adverse events before they occur, enhancing patient safety and quality of care.
Shaping the Future of Drug Safety Monitoring:
The integration of AI in pharmacovigilance literature is reshaping the future of drug safety monitoring:
Real-time Surveillance: AI-powered surveillance systems continuously monitor pharmacovigilance literature, regulatory databases, and real-world data streams for emerging safety signals. This real-time monitoring enables rapid response to safety concerns, minimizing the potential impact on patient health.
Personalized Medicine: AI-driven pharmacovigilance facilitates personalized medicine by analyzing individual patient characteristics, genetic factors, and treatment histories to predict drug responses and identify personalized safety profiles. This personalized approach enhances treatment efficacy while minimizing the risk of adverse events.
Cross-disciplinary Collaboration: AI fosters collaboration between pharmacovigilance experts, data scientists, healthcare providers, and regulatory agencies, driving innovation and knowledge exchange. Cross-disciplinary partnerships enable the development of AI-driven solutions tailored to the unique needs and challenges of pharmacovigilance.
Challenges and Considerations:
Despite its promise, the integration of AI in pharmacovigilance literature poses several challenges:
Data Quality and Bias: AI models are only as reliable as the data they are trained on. Ensuring the quality, diversity, and representativeness of pharmacovigilance data is essential to mitigate biases and improve the generalizability of AI-driven insights.
Interpretability and Transparency: AI algorithms often operate as "black boxes," making it challenging to interpret their decision-making processes. Enhancing the interpretability and transparency of AI models is critical for building trust and confidence in their recommendations.
Regulatory Compliance: Regulatory agencies impose stringent requirements for pharmacovigilance data analysis and reporting. Ensuring compliance with regulatory standards while leveraging AI technologies requires careful validation, documentation, and adherence to best practices.
Ethical and Legal Implications: AI-driven pharmacovigilance raises ethical and legal concerns regarding patient privacy, data security, and algorithmic accountability. Safeguarding patient data and ensuring compliance with data protection regulations are paramount to ethical AI implementation in pharmacovigilance.
Future Directions:
Looking ahead, several trends and developments are shaping the future of AI in pharmacovigilance literature:
Advancements in AI Technologies: Ongoing advancements in AI, including deep learning, reinforcement learning, and federated learning, will enhance the capabilities and performance of AI-driven pharmacovigilance solutions.
Integration with Real-world Evidence: Incorporating real-world evidence from electronic health records, wearable devices, and patient-reported outcomes will enrich pharmacovigilance data and enable more comprehensive safety assessments.
Ethical AI Governance: Establishing ethical frameworks, guidelines, and governance mechanisms for AI-driven pharmacovigilance is essential to ensure responsible and transparent use of AI technologies.
Patient-Centered Approaches: Empowering patients to actively participate in pharmacovigilance through patient-reported outcomes, mobile health applications, and social media engagement will enable a more patient-centered approach to drug safety monitoring.
Conclusion:
In conclusion, AI is in motion, revolutionizing pharmacovigilance literature with its transformative capabilities. By harnessing the power of AI-driven analytics, we can unlock valuable insights from vast volumes of data, ultimately advancing the field of pharmacovigilance and safeguarding public health. As we witness AI in action, we embark on a journey towards a future where drug safety monitoring is proactive, personalized, and powered by data-driven insights.
Comments